When re-thinking your loan origination process, it’s vital to update or to integrate a credit scoring solution.
In this article, we are going to provide you with the benefits of innovative credit scoring integration and having access to credit scoring analytics. Are there ways to improve your current approach to application management to avoid bad loans and improve the process? Is that true that without making the most of traditional and alternative credit scoring, you could find yourself overwhelmed by bad loans and fail to meet regulatory requirements? Let’s find out.
Integration of Various Credit Scoring Approaches
They say knowledge is power, and that’s precisely what a credit scoring system is. The credit scoring approaches and software have become a lot more sophisticated in the past few years. Leaving traditional statistical techniques in the past, the financial industry adopted AI and ML algorithms with intriguing definitions, like random forests, gradient boosting, or deep neural networks. It helped to expand the data types applicable for credit scoring models and AI-based decision-making.
Read also
4 Reasons to Consider AI-based Credit Scoring for Your Lending System
Better financial inclusion and accessibility of loans
A few years ago, World Bank stated that almost two billion adults worldwide didn’t have a basic bank account, while 200 million micro, small and medium enterprises (MSMEs) in emerging economies lacked financing due to being thin-filed or having not enough collateral. If that sounds surprising, imagine over 90% of adults in developing countries have difficulties financing debts at traditional financial institutions.
Alternative lenders are appearing just on time, while the absence of a credit history leads to blocked credit opportunities for potentially good borrowers.
Smart credit scoring that implements AI and ML allows collecting and analyzing a wider range of information about the borrower, thus increasing the chances to be assessed and approved.
Higher accuracy of underlying risk models
AI allows building better predictive models and, as a result, improves the credit risk assessment process and takes more accurate decisions. Credit services providers (CSPs) will be able to provide more competitive interest rates due to collective real-time alternative data, such as online transactions or mobile application data.
Automation and fast processing of loan applications
Digital Loan Origination Process: Integrity and AutomationThe implementation of innovative credit scoring models helps cut costs of loan decisions, loan monitoring, and overall operating costs. Quick data analysis means handling a wider range of loan data inputs, so it increases the number of end customers for whom lenders can measure the credit risk. Time savings happen due to automating repetitive tasks, and the assessment of real-time payments (for example, mobile operator, utility bills, and so on).
Improved customer experience
Due to versatile analytical capabilities and larger data sets analyzed, AI-based credit scoring models help lenders get deeper insights, offer personalized customer experience, and create relevant financial products.
Key Concerns about Innovative Credit Scoring Methods
- Data protection
- Discrimination of certain social groups
- Model interpretability
- Unintended bias due to learning on historical data
These issues can be solved individually with the software providers, there are certain tools that can help lenders deal with concerns and avoid risks.
Data Types Used for Credit Scoring
If you are still using only traditional data, you may consider innovative credit scoring approaches as they provide a better insight into borrowers’ creditworthiness.
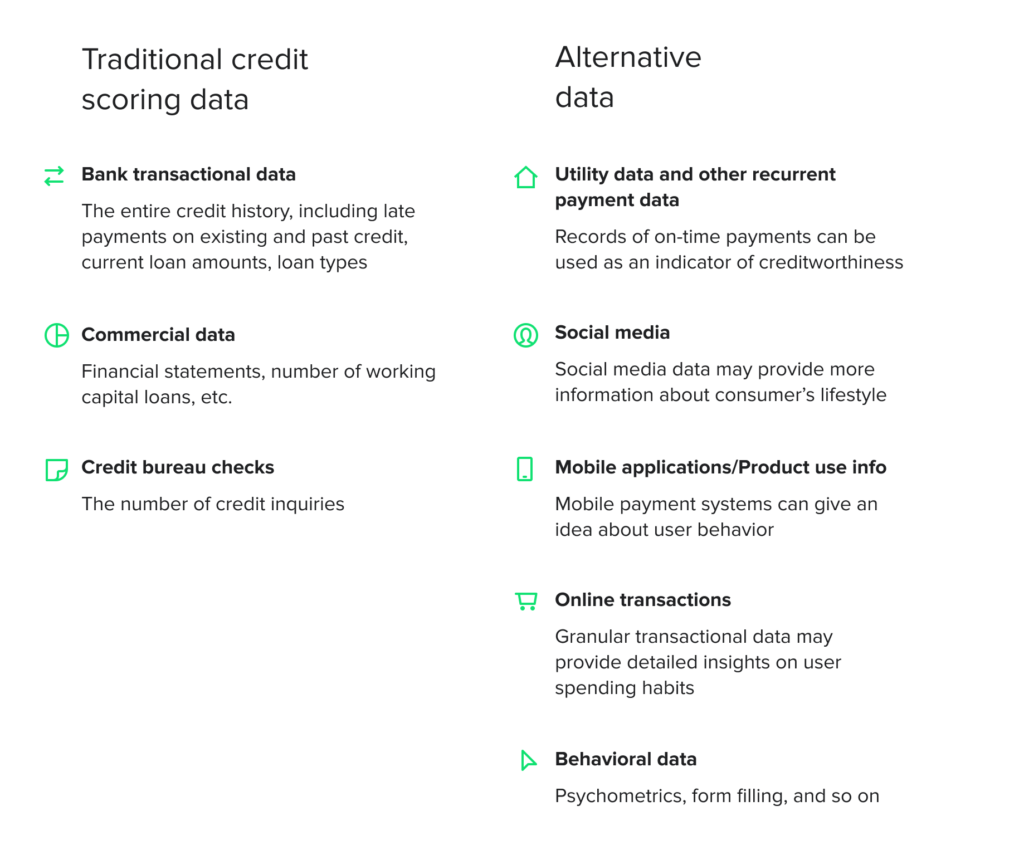
Why AI and ML in Credit Scoring Are Game-Changers?
Most used traditional credit scoring methods statistical discrimination and classification, including linear regression, logit, and probit models, discriminant analysis, and expert judgment-based models. Approximately 45 million Americans currently do not have a credit score.
How SME Lenders Can Tackle ‘Invisible Borrowers’ with Alternative DataTreditional scoring models can show quite good predictive power for customers with a long credit history. However, they can offer little flexibility and almost no opportunity no work with scarce, unstructured, or semistructured data. This way solely use of traditional scoring deprives businesses of valuable competitive advantages: use of digital data, big data, expanding to the underbanked markets. Tradition scoring is hardly able to fully meet financial market demands anymore.
AI and ML in credit scoring address operations traditionally requiring human involvement. Using these technologies computers can manage difficult tasks in processing and data pattern recognition, while the data can be of completely different types and from various sources.
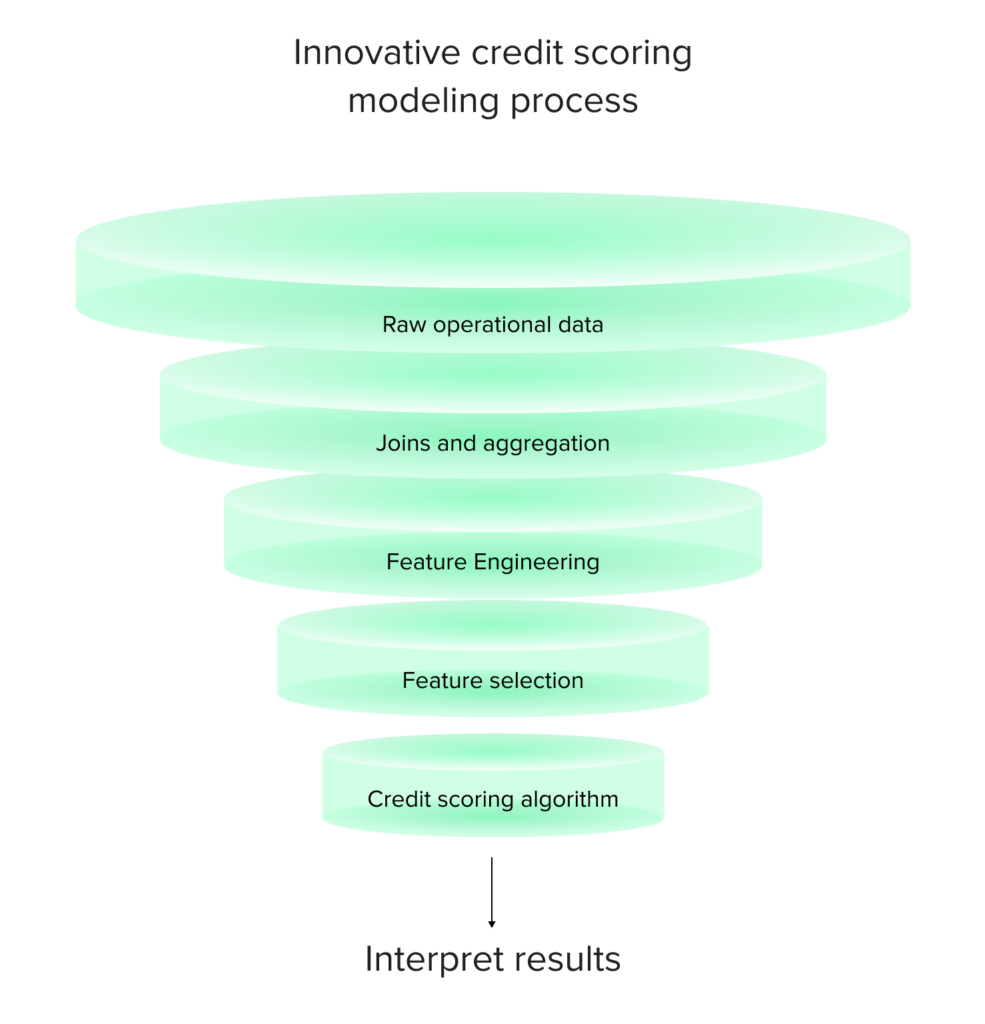
Here you can see a high-level demonstration of how machine learning algorithms are applied for credit scoring:
- The input of raw historical data
- Combining, joining, and aggregating the data
- Automated engineering
- Selecting the most useful features
- Using the ML algorithm to the training data set
- Interpreting, validating, and visualizing the credit scoring model
Innovative Credit Scoring Implementation: Real Results
Reduced NPLs: integrating a credit scoring model into your consumer loan origination system can help your business reduce non-performing loans by up to 30%.
Better loan portfolios: automated loan scoring integrated into a loan management platform improves the business effectiveness and boosts portfolio performance by 25-32%.
Higher customer satisfaction: by engaging a credit scoring model, you can assess your client’s ability to repay the loan faster based on their current circumstances. Giving them the answer, they need to make future financial decisions. Predictive AI makes all this possible, empowering you to make the right decision when you need them. The two times higher acceptance rate using this tool highlights the ability to make smarter loan decisions.
Compliance with regulatory requirements: loan providers are required to lend responsibly, and that means following both regulations and not taking unnecessary risks. Your loan origination software should be backed by the core tools it needs to automate onboarding — document reading software, data validation, and more — but adding credit scoring to the mix levels up your capabilities to perform safely and efficiently. Credit scoring ensures your business takes smart decisions and lends sensibly, reducing the risk to you and your client.
How to Choose the Top Loan Origination System for Your BusinessFlexibility: whether you are a bank, credit union, fintech, or another type of loan provider, innovative credit scoring software can be helpful in loan origination. It can work as part of a new software solution or a handy upgrade to your current technology stack, helping to assess the real credit history of your client in just a few simple clicks.
Read also
Credit Scoring Wrap Up
With the US-based TransUnion consumer credit forecast for 2021 predicting an up-tick in lending in the coming years, despite the economic dip of 2020 and the effects of the COVID-19 lockdown, there’s no better time than the present to start thinking about the future.
For future-thinking lenders, now is the time to get ready for the economic boom that will follow last year’s crash and prepare so that when healthy lending resumes, you are in the first place to deliver on sustainable loans and stand out from your competitors.