From OpenAI’s ChatGPT product to ObserveAI’s natural language tools, 2023 is the year that artificial intelligence stopped being science fiction and became a must-have tech solution. Over 55% of chief information officers (CIO) estimate that AI will continue to be a major area of investment this year. Meanwhile, 90% of respondents (Deloitte – Finance Innovation Survey 2023) say they’re already aware of AI initiatives in their company, 44% of which were initiated in finance departments. However, as past solutions have shown, AI is only as good as the data it’s given. Along with effective algorithms, AI data quality significantly affects how well any AI or ML solution will perform.
Poor data quality leads to big problems for AI and ML
So why does the data quality of AI matter? Any well-run organization today utilizes data to inform its business strategies. However, the accuracy of these decisions depends on the data they’re based on. The same goes for AI-analyzed data, except on a much grander scale.
Amazon’s infamous AI recruitment tool and Google’s AI bias are just some of the most well-known examples of AI solutions gone wrong. And if some of the world’s largest tech companies are noting challenges, it’s time to pay attention. As with every novel solution, some issues are bound to crop up along the way. When it comes to AI, data quality is often one of the first.
For AI solutions to work, they need to be accurate, and for that, they need accurate, clean data. Failure to manage data processes effectively can lead to:
- Inaccurate predictions, wherein data quality leads the AI to make low-accuracy predictions, which have a direct impact on business decision-making.
- Data breaches and security risks are caused by inappropriate handling of data or lack of security, which lead to financial and reputation risks.
- Inefficient use of time, money, and resources are spent following up on unreliable data, over time this can become costly to the business as data scientists need to take extra care cleaning and validating data.
- Limited scalability, when initial problems with data happen, this can lead to issues in scaling the software and deploying it effectively.
- Inconsistent data makes it almost impossible for the AI to make accurate predictions due to a lack of information.
- Low user rate adoption results from a lack of trust in an AI tool and users failing to adopt it, meaning that data accuracy is not trusted.
AI, data quality, and credit scoring
How AI is Used in Fintech in 2023: Top 8 Use CasesAs AI expands its realms of influence, one area where it has found its place is in credit scoring. Seeking to replace the more traditional methods of evaluating creditworthiness, such as the aging FICO, lending organizations are turning to AI-backed credit scoring. These tools draw upon a variety of non-traditional data to allow companies to access new types of borrowers more accurately.
AI models utilize available data to make predictions. And, as previously discussed, technology is only as good as the data that powers it, making the data quality for AI and ML solutions a top priority. These three steps are designed to improve AI data quality by solving some common AI data challenges:
No. 1: Create a strategy to improve your data quality in AI and ML
Approaching data management without a plan can be a lot like going on a road trip without a map. You can muddle your way through it, but the results will always be substandard and filled with unnecessary stress. Preparing in advance allows you to take a competitive advantage and ensure you improve AI data quality and its results.
- Start by defining the data sources and elements you need to gather.
- Establish data management processes drawing from best practices. This should always include data acquisition, storage, and distribution strategies as data security becomes ever more important.
- Outline document data management policies and procedures to allow your team to stay on track when managing data.
Combined, this not only allows your team to effectively and securely handle data, but also to use it effectively to ensure accurate solutions.
No.2: Identifying and addressing your AI machine learning data quality issues head-on
How AI Supports Inclusive Lending on the Way to Fairer CreditsIssues are bound to happen along the way, but burying your head in the sand is never a good strategy in business. The same goes for working AI-based solutions. Quality and strategy are key. That is why identifying and dealing with any and all data quality issues early on is a priority. Start by:
- Cleaning your data to ensure that it’s up to quality standards
- Conducting regular AI data quality assessments to identify any issues or worrying trends, such as bias
- Making use of data profiling tools to determine patterns and consolidate data more efficiently
- Establishing data quality metrics and targets that will inform data gathering and storage now and in the future.
By undertaking these actions, the data used by your AI model will be cleaned, leading to more accurate predictions.
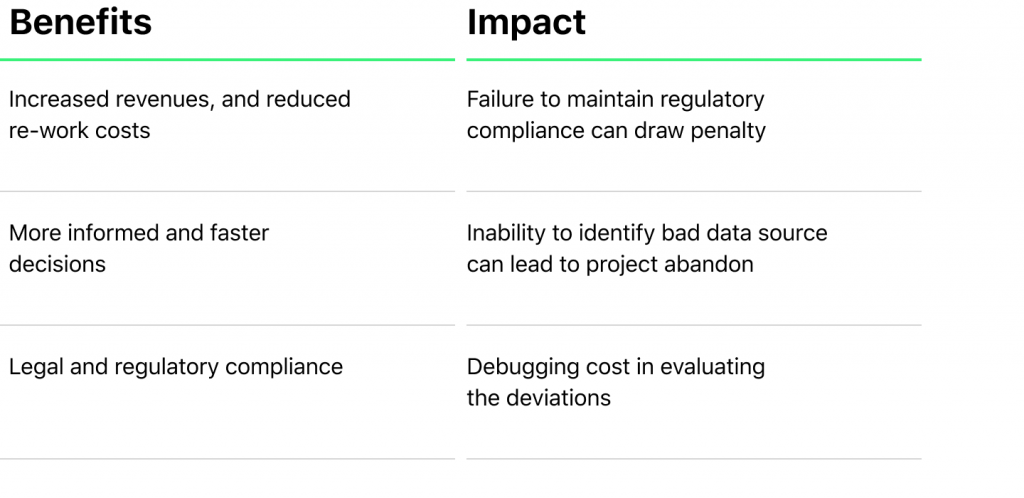
No. 3: Develop and implement wider data governance policies
Data is set to be a significant part of our lives for the common future, and it would be almost foolish to believe that current data management strategies can meet future demand. Instead, wider organizational change is needed. While necessary, it is often not as scary as it first appears when dealing with data quantity.
- Onboard the right team members to manage the process
- Establish a data governance framework to manage expansion
- Allow this team to manage, update and regulate internal data policies
- Ensure that you remain compliant through the process, especially when dealing with financial data.
Along the quality of AI with more data, organizations are often concerned with how they will handle the amount of data in their grasp. Having data governance practices in place early on can alleviate some of these concerns and lead to better solutions down the line.
Don’t let AI data quality sabotage your business plans
Data is growing. Estimates suggest that in 2023, there is around 120 zettabytes of data, meaning, that no matter your specific area of expertise, data analytics is already seeping into your business decisions in some shape or form. AI, especially when used for credit scoring, can prove an effective solution for dealing with large quantities of data and accurately, if done correctly. AI data quality plays a huge part in making this a reality.
Early onboarders and adopters of the technology can seek to take a competitive advantage and deliver better quality to their consumers. Research shows that although interest in harnessing AI solutions is high, the majority (59%) of solutions are considered low maturity, with only 6% indicating high maturity, indicating a market opportunity for companies seeking an innovative approach.
Not sure which steps to take when managing your data to ensure its quality and accuracy? Get in touch with the team at HES FinTech. We are the AI credit scoring experts on hand to walk you through the pitfalls of data to deliver more effective solutions that match your business needs.